
Sivic et al. use boosting orientation-based features to obtain training samples for their face detector. Lee et al. use a variant of eigentracking to obtain the training sequence for face recognition and tracking. Nair and Clark use motion detection to obtain the initial training set. Levin et al. start with a small set of hand labelled data and generate additional labelled examples by applying co-training of two classifiers. Then the classifier is applied on a training sequence, and the detected patches are added to the previous set of examples. Existing approaches to minimise the labelling effort use a classifier which is trained in a small number of examples. While a lot of research has been focused on efficient detectors and classifiers, little attention has been paid to efficiently labelling and acquiring suitable training data. These applications include visual surveillance , and object tracking . Segmentation is a pre-processing step in many computer vision applications. However, for the accurate analysis of the hand’s properties, a suitable segmentation that separates the object of interest from the background is needed. Since this method is too complicated to implement, the most widespread alternative is the feature-based method where features such as the geometric properties of the hand can be analysed using either Neural Networks (NNs) or stochastic models such as Hidden Markov Models (HMMs) .
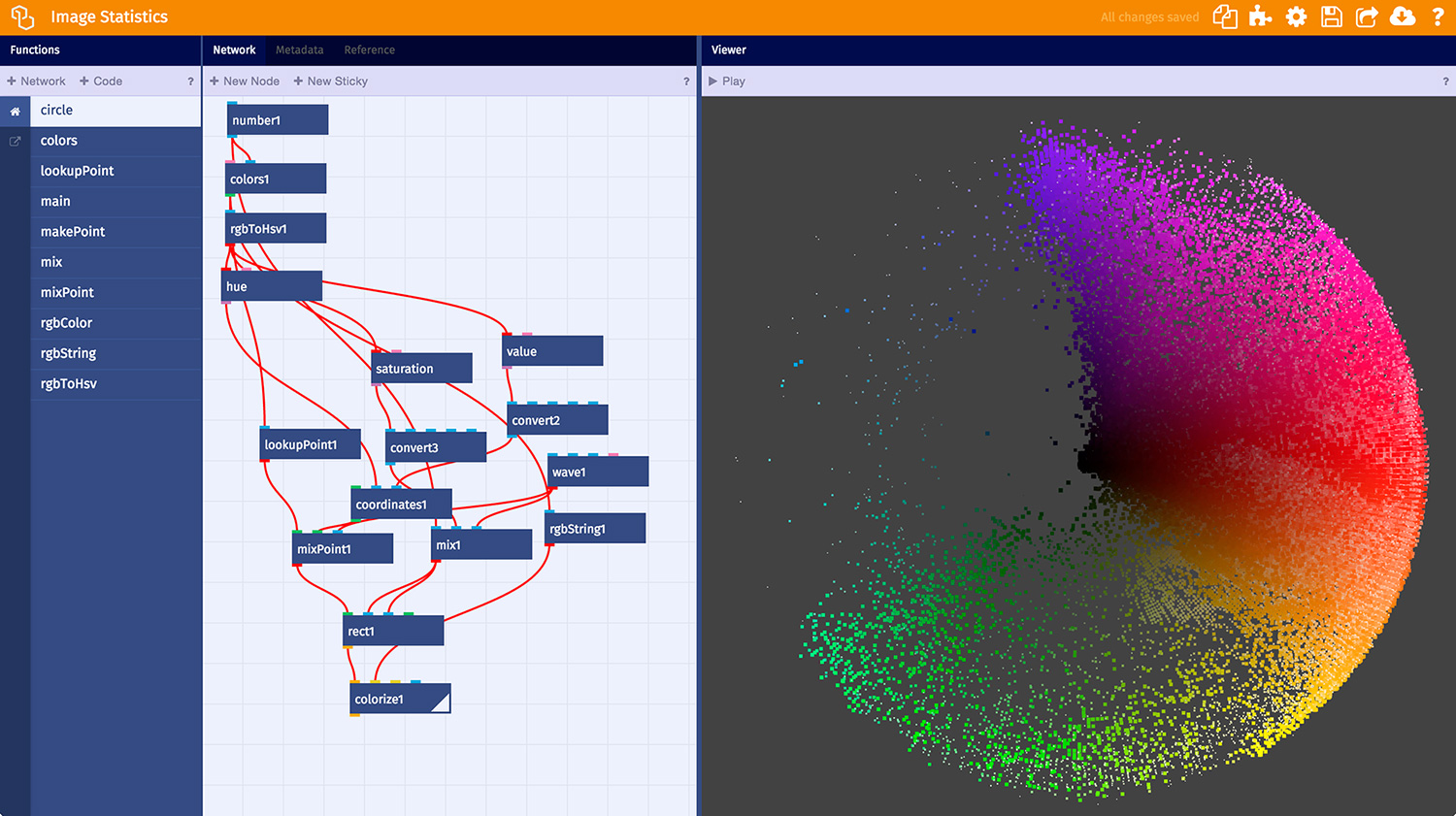
The main drawback of this method is that it requires massive calculations which makes it unrealistic for real-time implementation.
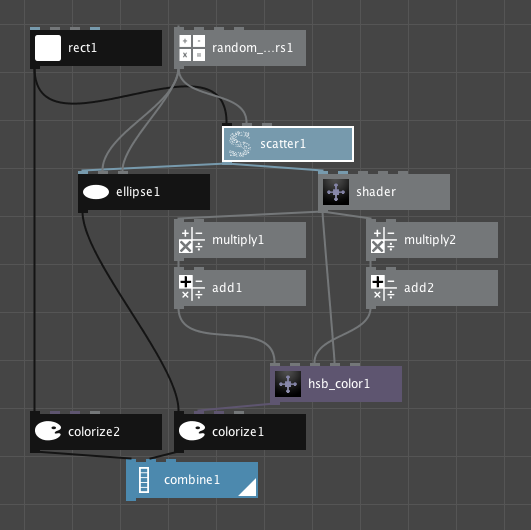
Systems that follow a model-based method require an accurate 3D model that captures efficiently the hand’s high Degrees of Freedom (DOF) articulation and elasticity.
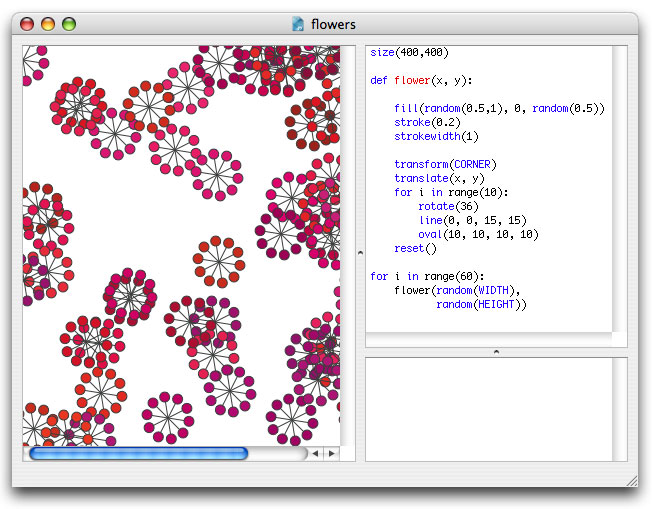
The images captured of hand gestures, which are effectively a 2D projection of a 3D object, can become very complex for any recognition system.
